How Maritime Shipping Companies Can Leverage Performance Software to Increase Commercial Returns
- delauno
- Jun 21, 2023
- 7 min read
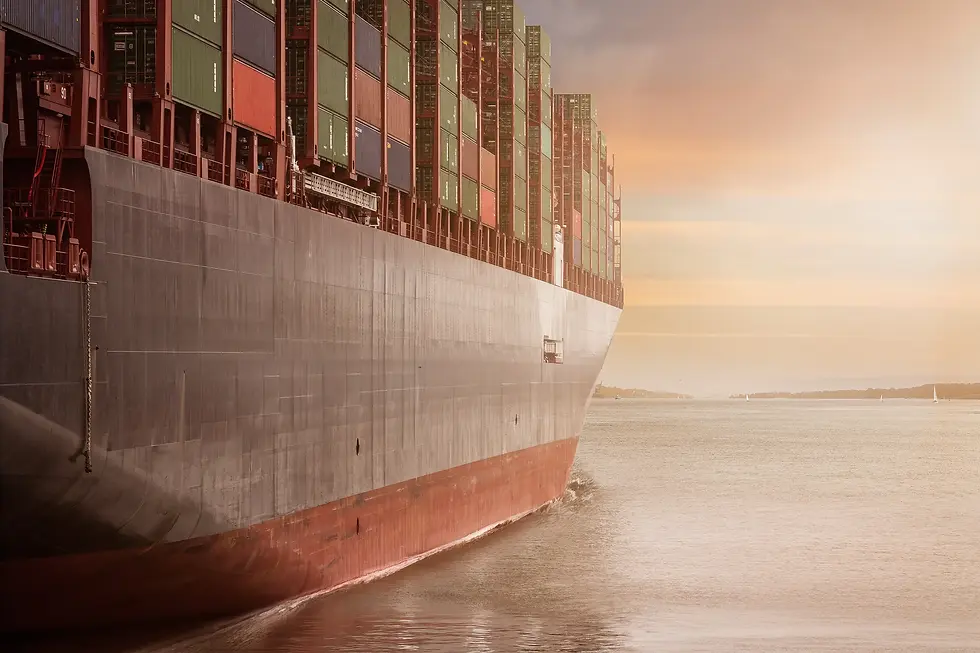
The maritime shipping business model has evolved in recent years. Intensifying global competition, heightened macroeconomic volatility, stringent environmental regulations, stiffer capital access requirements, and a recurring pattern of black swan events have all eroded the foundation of the patient asset-play model. A new paradigm focused on generating more consistent returns by improving operational efficiency is gaining recognition as the industry’s future.
In acceptance of this new paradigm, owners of all sizes have accelerated their attempts to leverage more data throughout their operations. The result has been both an increase in the amount of data required from each vessel, as well as an increase in the number of stakeholders involved in evaluating fleet performance data. In many cases, companies have appointed a dedicated performance leader, or created entirely new performance teams.
But in the process of trying to improve operational efficiency, major decision-making challenges have hindered the ability of many teams to move the needle. The more that teams have tried to aggregate and analyze different types of data, the more they’ve discovered the challenges of establishing consistency across so many different data sets, as well as converting that disparate data into actionable insights. Data-intelligent decision-support is the critical missing piece in the process to achieving better commercial outcomes. This is precisely where a maritime-specific voyage performance software solution can play an invaluable role.
Given the unique nature of the maritime shipping business, and the robust capabilities required to convert better operations into higher returns, it is important to understand what this performance software should be able to tackle. The following essay unpacks the four key challenges that shipping businesses face in the process of taking a data-driven approach to improving operational efficiency, as well as how the right software will solve those problems in a way that translates into what most shipping companies desire — more profitable voyages and higher asset yield.
1. Better Performance Requires Unified Data Across Teams
A key pain point in the shipping industry is the operational inefficiency resulting from an inability to unify, standardize and evaluate data coming from the various systems used by different teams. Legacy software platforms are a big part of the challenge. Deployed during the first wave of shipping digitalization, these technical, weather-routing, operational, and commercial platforms were designed to address specific pain points related to the monitoring, navigating, tracking or fixing responsibilities of each individual unit. What they weren’t designed to do was interoperate with other point systems to facilitate greater transparency, and tighter cross-team data sharing. And the inefficiencies resulting from these disconnected systems can have a real financial consequence across major parts of the underlying business. For example:
Commercial teams routinely fix vessels using outdated Speed/Consumption curves due to lack of access to another team’s performance system, driving further uncertainty into the pre-voyage planning process — which can leak money.
Operations teams swivel-chair between multiple systems, but struggle to quickly identify which vessels are overconsuming against their charter party agreements (while factoring in weather), or see which ships are sailing outside of ordered speed, or quantify the amount of performance deviation occurring between sister vessels — all of which can leak money.
Technical teams struggle to quantify the performance impact of a hull or propeller cleaning, or understand when there’s consistent, unnecessary generator consumption during passage, or visualize and understand the impact that fuel blends are having on vessel performance, — all of which can leak money.
Performance analysts spend hundreds of hours downloading, standardizing and manually entering disparate data into spreadsheets, and then manually updating that data for any corrections, instead of developing actual analysis and recommendations that can drive better outcomes — which can leak money.
Executive teams struggle to understand fleet performance in real-time, limiting their ability to actively monitor or adjust KPIs across teams to drive accountability and behavioral change.
The list goes on and on. Managing performance based on siloed systems can cause financial leakage before, during and after a voyage.
Connected Teams Require Connected Intelligence: For this reason, the ability to programmatically collect, operationalize, and visualize the relevant data coming in various formats from various sources, all into one place is a prerequisite for any performance platform. Unifying and contextualizing commercial, weather, historical, noon, and vessel IoT data enables shipping companies to gain a comprehensive, real-time understanding of performance vis-à-vis commercial expectations. In the modern world of multi-team collaboration, all teams must be able to visualize and leverage the same data across their entire fleet, even though each team will continue using that data to evaluate and optimize for their own, respective, KPIs.
2. Higher Returns Require Higher Frequency Data
To drive better performance outcomes, high frequency vessel data is a critical ingredient. The limitations of Noon reports as a sole source for vessel performance data (which are both infrequent and error prone) is well documented. And yet, in a world where any runner’s smart watch collects more health data points in a day than many operations teams receive from a vessel in a month, static Noon data remains the bedrock upon which most technical, operations, commercial and performance teams determine the health and capabilities of each and every vessel within their fleet.
Defenders of Noons argue that the hardware CAPEX and installation costs are an unnecessary expense if Noon data can reach a high enough quality threshold, and be combined with additional data about the vessel’s voyage behavior (e.g. AIS movement or generic performance benchmarks). Taken together, the resulting analysis should be sufficient for seasoned managers to evaluate performance trends and run their business, or so the thinking goes.
However, multiple studies have shown that leveraging historical trends based upon data of inconsistent quality isn’t sufficient to consistently improve commercial voyage outcomes because dynamic environments require analysis based upon dynamic data. For owners seeking better returns, they’ll need to invest in something that Noons, as a stand alone data source, will never provide, and that’s high frequency vessel data — specifically shaft power data from the torque meter, fuel flow data from the flow meters, and any cargo data required to evaluate cargo performance.
For owners that have awakened to the value of telemetry data, the right software solution will be able to programmatically ingest data from any hardware provider. This gives the owner ultimate flexibility in the process of deciding the best OEM partnership(s) for their business.
3. The Promise of Machine Learning: Professional intuition supported by advanced data science
The purpose of a vessel performance model is to provide an accurate description of how a vessel will perform in any nautical environment. And yet, teams routinely leverage speed/consumption curves that are unable to account for the impact of weather, sea-state, and draft. Recognizing these deficiencies, many of these teams have tried to compensate by automatically increasing voyage consumption estimates by up to 15% per knot as a “weather buffer”. Not only does this buffer throw off pre-voyage TCE estimates, there’s also a domino effect that stifles the ability to predict, and thus optimize the financial outcome of the next leg or voyage.
But that’s not the only weakness in these models. Two other major issues often exist:
The models are typically built in Excel, PowerBI, Matlab, or some other non-maritime-specific business analytics tool, and thus are not easily able to integrate with different data sources and automatically update themselves.
They’re almost universally unable to automatically learn from historical data patterns to the point of developing predictive inference.
Solving all of these weaknesses is necessary to develop a comprehensive, accurate, and bespoke understanding of a ship’s performance capabilities. To do this, a solution must utilize the dynamic analytical power of Machine Learning.
By combining high frequency vessel telemetry data, noon, weather and sea-state data, and then framing algorithms with the physical principles of naval architecture, the right solution should turn the torrent of technical and performance data into powerful insights, and then refine those insights until they can become value-driving, confidence-weighted recommendations. For example:
ROI-Based Conditional Maintenance: No longer do technical teams have to rely on pre-scheduled anti-fouling maintenance events such as a hull cleaning without a clear understanding of how fouling is affecting vessel performance. Furthermore, machine learning also enables the ability to quantify the efficacy of the anti-fouling event in terms of vessel efficiency gained over a period of time, translating the return directly into both consumption and emissions reduction, and enabling greater business decision-making in the future.
Better Benchmarks: A new understanding of performance post dry-dock can act as a more useful benchmark versus the sea trials that may be a stale and irrelevant representation of the vessel’s performance. In addition, machine learning-based performance models can detect and quantify the differences in performance between sister vessels, enabling commercial and operations teams to make more informed chartering and operating decisions.
Intelligent Alerting: Vessel telemetry data tells two performance stories: one story is about the performance of the vessel, the other is about the performance of the sensors themselves. The right platform will have the ability to read and understand both stories, and translate those understandings into intelligent alerting for each. Identifying that a vessel has reached a degradation threshold only matters if the underlying data is valid, and machine learning enables the rapid and accurate detection of both.
4. Dual Outcome-Centered Voyage Optimizations
When faced with the question of whether teams would rather have the ability to improve consumption efficiency or increase voyage profitability, the answer from shipping executives is almost always: both.
Even though the industry has ample reason to focus on improving energy efficiency, owners know that they can never lose sight of commercial returns. For this reason, the right performance platform will offer the ability to optimize voyages for consumption efficiency in some cases, and net profitability in others. This is no small feat given the complex variables that influence these different desired outcomes.
Net Profit Optimization: For example, optimizing voyages in the spot market requires the ability to take an up-to-date, weather-normalized understanding of a vessel’s performance capabilities, and combine that understanding with the expected daily voyage revenues, costs, voyage duration, expected weather and route. That’s the first step. Then, as the ship sets sail, voyage optimization also requires the ability to run simulations that dynamically account for each of these fluidly changing variables, while also introducing and factoring a new one into the analysis: the opportunity cost of “the market,” or value of the next fixture. Once the financial implications of both the current, and the potential next voyage are accounted for, only then is it possible to ascertain the best way to run the voyage that maximizes net profit.
Flexible ETA Optimization: On the other side, to optimize for consumption efficiency, the market dynamics play a lesser role. But the ability to run dynamic simulations based upon flexible ETAs (among other aforementioned variables), and then translate the results into an updated RPM or power recommendation requires its own nuanced approach.
Measurement of Success: The process doesn’t end there. For each of these multifactorial analyses, a clear understanding of how to measure the performance of the voyage is required. In other words, did the voyage outperform because of better than expected weather, or because the software did its job? Or both? To answer these questions, a counterfactual methodology must be established before every voyage so that accurate comparisons can be derived from voyage outcomes. The right solution provider must understand how to apply this methodology to improve the success rate of future voyages.
In conclusion, shipping companies that are serious about squeezing more yield from their vessels must get serious about investing in the right performance software. For owners in need of additional support in their evaluation process, let’s connect.
Comments