“Phygital” is Retail’s Next Frontier, LaunchDarkly’s Experimentation Engine Helps Chart the Path
- delauno
- Jun 16, 2023
- 5 min read
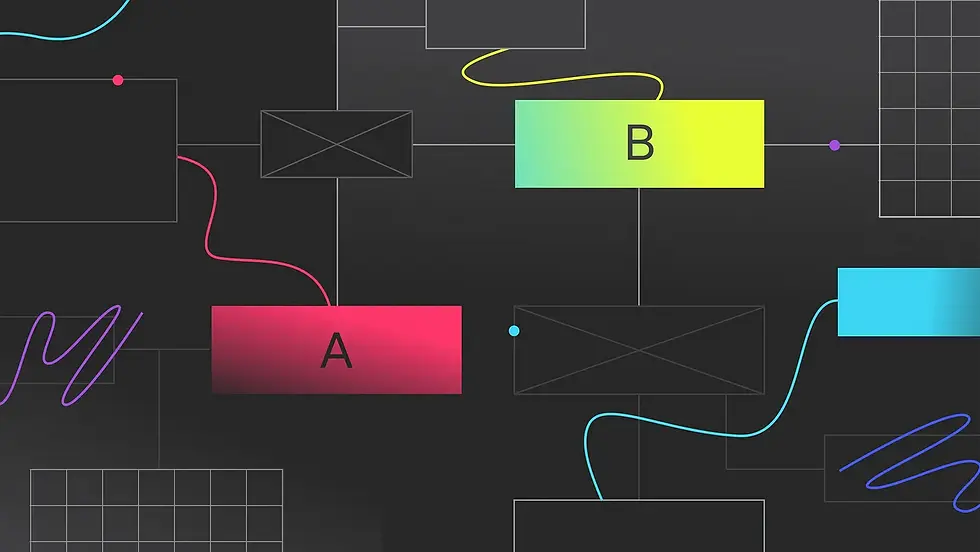
There’s never been a more exciting time for digital product leaders in the retail industry. Today’s leaders are racing to create a shopping experience that, for the first time, synergizes the physical in-store experience with omnichannel digital. “Phygital”, as it’s called, has been heralded as the industry’s next frontier.
Phygital’s promise can be seen in hybrid shopping patterns that emerged from the pandemic. For example, consumers are increasingly spending more time researching purchases online, spending less time in-store, yet buying more on impulse once inside and engaged with the brand. Patterns like this suggest a clear opportunity for retailers to strengthen both customer loyalty and store operating efficiency by delivering highly personalized, elegantly blended shopping experiences.
But what are the features and service components (or combination thereof) that will best drive conversion? Phygital’s emergent nature means that proven play books don’t exist. And evolving consumer expectations make the concept of “personalization” a moving target.
With so much potential yet so much complexity, and no proven roadmaps, there are only two paths that can successfully lead to the creation of high-impact Phygital experiences: luck, and consistent experimentation.
Product leaders that choose the latter will need the ability to routinely test and measure new features based on two separate but connected lenses:
Product Performance: Does the new or improved feature achieve its functionality and stability KPIs (e.g. page load times, API response times, algorithm performance, cross platform dependencies, etc.)?
Business Performance: Does this new or improved feature improve conversion and / or other primary and secondary metrics?
This depth of experimentation capability can’t be found in marketing focused experimentation tools. New features are being built on an increasingly complex and interdependent tech stack. Today’s product leaders need a single platform from which they can:
Validate that a new feature has been well engineered before it’s ready for experimentation
Run experiments across the full retail tech stack without impacting application performance
Precisely deliver those experiments to granularly defined audiences, without any slippage
Receive results in the form of easy-to-understand decision support data
Facilitate multi-team collaboration in the experiment process
Iterate on experiments quickly through configurable workflows and reusable components
And if necessary, seamlessly translate experiment feedback back into the product engineering cycle
At a high level, this is why product leaders throughout the retail industry are rapidly turning to LauchDarkly’s feature management-based experimentation engine. Diving deeper, it’s because our all-in-one platform enables these leaders and their teams to leverage six core functions that facilitate a “test-and-learn” culture, and consistently lead to better experimentation outcomes:
1. Safely validate feature readiness through zero latency testing in production
A prerequisite to running experiments on any new feature or upgrade is ensuring that the new feature or upgrade is functioning properly from an engineering standpoint (the first lens). As such, the first way that LaunchDarkly supports product leaders is by empowering them to safely validate product performance in partnership with their engineering teams by leveraging feature flags.
For those less familiar, feature flags are a software risk mitigation tool that can instantaneously turn on or off any piece of software that’s been “wrapped in a flag”, without the need for a time-consuming application reset or deployment of new code.
LaunchDarkly’s category leading feature management platform amplifies the power of feature flags by enabling product and engineering teams to deploy a new or upgraded feature in a live production environment, exclusively for pre-defined audiences, as a way of testing a new feature’s performance. A feature that works properly during an initial review can be further rolled out in controlled stages to additional audiences until that feature is proven ready for full release to the general public.
On the other hand, the feature can be rolled back instantly by turning off the flag if any misbehavior is observed during any of the controlled group evaluations. After developer teams fix the issues, the same flag can reactivate the same controlled deployment process until the feature is ready for full production rollout.
2. Testing in production enabled across the full retail stack
LaunchDarkly powers the controlled production-testing process for features written in 24 client-side and server-side development languages and frameworks. This is especially important for product leaders due to the ever-growing number of applications involved in supporting the user experience throughout the retail stack. For example:
A new search algorithm built in Python
A new pricing elasticity program written in Ruby
New modals written in JavaScript
New geo-based mobile app offers written in both Swift and Kotlin
Various microservices storing user data
Database infrastructure written in .NET
Each of these, and many other examples can all be performance-tested in a controlled manner by using feature flags. And by leveraging LaunchDarkly to perform live-environment testing, product and engineering teams can validate product performance faster and safer than ever before.
3. Experiments tied to metric-based flags, built with an easy workflow, and delivered to granular audience segments
Once the engineering performance of a new feature has been established, product leaders can now turn to the second lens: business performance.
With feature flags already acting as a control point for validating the functionality of new features, each of these flags can now act as the centerpiece of an efficient experiment workflow consisting of a few simple steps:
Establish a hypothesis to be tested
Assign metrics (either standard or imported) to be measured when a corresponding flag is activated
Determine the type and complexity of experiment (LaunchDarkly’s module supports a broad swath of experiment types and complexity levels to meet the unique needs of different teams)
Determine the experiment audience(s) (segments can be based on any identifiable attribute)
Determine workflow rules (duration / review and sign-off process requirements / experiment dependencies / etc.)
Run it!
4. Full stack experiments executed with negligible site or application performance impact
Marketing oriented experimentation tools often lack the ability to deliver experiments across the full retail tech stack, but that’s not their only weakness. Another issue is that experiments executed through these tools often degrade website performance. And stalled page load speeds or site flickers don’t just harm the user experience, they can distort experiment results as well.
LaunchDarkly’s modern platform architecture solves this performance issue through the ability to bring payloads, updates, and evaluations to the edge of our own globally distributed Content Delivery Network (CDN). This means that experiment changes delivered to the test group(s) can occur in 25 milliseconds or less. For context, that’s about a quarter of the time it takes to blink an eye. The outcome of this advanced, global distribution network is that experiments can run without performance degradation, whether the user has high speed bandwidth in New York, or is experiencing limited bandwidth in New Delhi.
5. High-confidence results, based upon standardized, referenceable data science, delivered through easy-to-understand performance dashboards
Higher granularity of user segments often equates to smaller-than-desired data sets. For this reason, the LaunchDarkly experimentation engine relies upon Bayesian statistics, which can squeeze higher confidence outcomes on smaller data sets.
What’s more, results are clearly defined and visualized inside elegant dashboards so that audiences lacking deep knowledge of statistical significance, p-values, or sample size calculations can still easily understand experiment outcomes.
And not only is the underlying data referenceable, it can also be exported for additional analysis by an in-house data science team.
6. Close the loop by rolling out the winner, or by returning the feature for modification
A successful experiment won’t always be a winning experiment. Sometimes, customer feedback data will point to necessary feature alterations. In these cases, LaunchDarkly’s closed loop system provides product leaders the ability to apply the same feature flagging process to rollback the experiment and feature, and return it to the original build process.
Furthermore, the closed-loop process and enterprise scalability means that different teams can all leverage the platform for their own product development and experimentation needs, without ever stepping on each other’s toes, thus enabling a culture of experimentation.
Conclusion:
Delivering successful Phygital experiences requires the ability to continuously validate both the engineering performance and business KPIs of any new feature. For this to happen, experimentation must be fused to feature development, and democratized across all teams. This is the promise of LaunchDarkly, and the reason why retail industry product leaders are entrusting us to help improve both their product outcomes, and their “test-and-learn” culture.
Comments